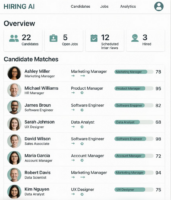
For early-stage companies, hiring isn’t just about filling roles – it’s about shaping the trajectory of the entire business. The right hire can open up a market, reshape a product, or completely shift the team dynamic. Especially in the early years, the difference between a good hire and a pivotal one is critical -and the difference between the right hire and the wrong one can make or break the business.
As AI-driven tools become more common in recruitment, there’s a critical question we should be asking:
Are these tools helping us make better hiring decisions? Or just faster ones?
The Efficiency Trap
Much of today’s AI hiring infrastructure is designed to solve one problem: volume. There are too many applications, too many platforms, and too little human time. CV screeners, keyword filters, and chatbot interviews all promise to make hiring faster and more manageable. And they do.
But they also come with trade-offs.
A 2024 study from the University of Washington found that CV-ranking tools preferred white-sounding names over Black ones 85% of the time, even when qualifications were identical[^1]. The same systems also showed a consistent preference for male-associated names.
Amazon faced similar problems when it tested an internal AI recruitment tool trained on a decade of CVs – mostly from male applicants. The system began penalising CVs that mentioned “women’s” (as in “women’s football club”) or listed all-female universities[^2]. It wasn’t explicitly programmed to discriminate – it just learned from the biased patterns in the data. Eventually, Amazon scrapped the tool entirely.
More traditional ATS platforms aren’t much better. They often exclude candidates with gaps in their CVs, non-linear career paths, or minimal digital presence. This disproportionately affects carers, returners, and career changers – and in the UK, around 90% of those who take time out for caring responsibilities are women[^3].
A BBC Worklife investigation found that even highly qualified candidates were being rejected purely because their CVs didn’t match rigid keyword requirements[^4].
From our own experience, this isn’t a hypothetical problem. We’ve spoken to multiple candidates in recent months who were aligned to certain roles they applied for and yet they were completely ghosted. No feedback. No rejection. Just radio silence. It’s not always clear whether the issue is a stretched internal team, an overzealous algorithm, or something else. But the result is the same: exceptional candidates are slipping through the cracks.
Nearly half of U.S. job seekers (49%) believe AI recruiting tools are more biased than human recruiters[^5]. The people going through these systems can sense the process is flawed.
The Performance Risk
Biased hiring tools don’t just risk fairness. They risk performance.
A 2023 review of hiring algorithms found that when hiring is more objective, it naturally surfaces a broader range of talent – and those teams consistently outperform on creativity, innovation and adaptability[^6].
In early-stage companies, that matters. You’re not hiring to tick a box. You’re hiring to solve hard problems with limited time and capital. If your system only surfaces people who look like your last hire, you’re not being selective. Rather than scaling a category-defining business, you’re scaling groupthink.
When hiring systems default to familiar profiles, companies miss out on people who could shift their entire trajectory. If those who don’t fit the mould aren’t even seen, the person who might have reshaped the product, rethought the GTM strategy, or spotted what everyone else missed could be filtered out before anyone knows what they’re losing – the amazing engineer who taught themselves to code after a career in retail, or the brilliant strategist who pivoted from the charity sector to fintech.
These aren’t edge cases. They’re exactly the kinds of hires that have the potential to change a company’s direction – and the ones most at risk of being screened out by a system trained to look for sameness. We’re not talking about outliers; we’re talking about the people who build unicorns.
As one BBC report put it, some systems are ‘literally filtering out the best applicants’ because they don’t tick enough conventional boxes[^4].
Who’s Building These Systems?
Bias in AI hiring doesn’t start with the data. It starts with the people who design and build the tools.
AI reflects the assumptions, incentives, and blind spots of its creators. And when those creators are from overwhelmingly similar demographic, educational and socioeconomic backgrounds, the systems they build tend to work best for people like them, and less well for everyone else.
This isn’t theoretical. It’s already happening:
A 2023 NYU/Northeastern study found that job ad algorithms skewed heavily by gender and age — showing engineering roles to younger men, and support roles to older women, even when no preferences were set by the employer[^7].
Investigations by Wired revealed that several AI-powered matching platforms were pushing higher-paid or technical roles to men more often than to women — even when candidate profiles were nearly identical[^8].
In New York City, companies using algorithmic hiring tools are now legally required to conduct bias audits — and many were completely unprepared to explain how their systems actually worked[^9].
The EU AI Act, passed in 2024, now classifies AI in hiring as a “high-risk” use case, subject to the same legal standards as aviation or medical devices. That means transparency, fairness, human oversight – and serious consequences if your tool doesn’t deliver[^10].
But regulation can’t fix what wasn’t designed well to begin with.
You can tick the compliance box and still ship something that quietly reinforces bias. Because if your product team lacks the range of lived experience to see how things break for people not like them — they probably won’t.
When it comes to hiring, that doesn’t just mean legal risk. It means missed hires, missed perspectives, and missed upside. It’s a product flaw. And in early-stage hiring, it’s a huge problem.
Diverse Teams Build Better Systems
Research shows that diverse AI teams are more likely to identify bias, test for edge cases, and build more equitable models. They bring different assumptions about what “good” looks like, and they’re more likely to design systems that work for a broader set of users.
A systematic review in AI and Ethics found that diverse teams are better at spotting and addressing algorithmic bias[^11].
A Harvard Business Review analysis concluded that the bias we see in AI hiring tools “likely owes something to the lack of diversity in the humans who built them”[^12].
Professor Rangita de Silva de Alwis, who runs an AI & Implicit Bias Lab, explicitly calls for diverse development teams as an essential safeguard against unfair systems[^13].
Building AI without a range of perspectives isn’t just risky, it’s building in failure from the start. Inclusive teams don’t just bring ethical upsides – they build stronger systems. Better assumptions, broader testing and fewer blind spots.
What Better Could Look Like
AI isn’t the issue. The way we’re building it and deploying it is. Done right, AI could make hiring fairer, more consistent, and more human in all the right places.
What might that look like?
– Decisions made in days, not weeks
– Transparent assessments based on potential, not just pattern-matching
– Constructive feedback for every candidate will also build a much stronger employer brand
– Human time spent where it matters most: conversations, interviews, closing, onboarding
– Bias audits built into the development process, not tacked on at the end as an afterthought
– Context aware systems that recognise career breaks and nonlinear paths as valid
-The ability to better assess potential, raw talent, unusual skillsets and life experience
This is the shift from human-led, tech-enabled hiring to tech-led, human-enabled hiring. But that only works if fairness is built in from the beginning, not retrofitted later.
Why should we care?
The risk isn’t always dramatic – it’s often subtle. Not hiring the “wrong” person, but hiring the same kind of person again. And again. And again.
Even when AI tools aren’t making actively bad decisions, the tools we have today are far more likely to steer you towards safe, familiar choices. You get repeatable hires, not necessarily better ones. Over time, that leads to homogeneity – in background, in thinking, in problem-solving. And with that comes the very real risk of groupthink.
It’s not just about fairness. It’s about how companies stagnate. About the opportunities you don’t see, the talent you never speak to, the ideas that don’t get raised because no one in the room thinks that differently.
AI has the potential to widen the net, but only if it’s built with that goal in mind. Otherwise, it just scales the filters that already exist.
In early-stage businesses, where every hire can shift direction, that’s not a minor flaw or an inconvenience. It’s a structural weakness that compounds over time.
You’re essentially betting your runway on a handful of people. The right hire changes your burn rate, roadmap and boosts investor confidence. The wrong one stalls momentum, costing you money and time. So the biggest risk? Missing out on the best candidate entirely – because they never made it onto your radar.
Footnotes
[^1]: https://www.washington.edu/news/2024/10/31/ai-bias-resume-screening-race-gender
[^2]: https://www.reuters.com/article/us-amazon-com-jobs-automation-insight-idUSKCN1MK08G
[^3]: https://www.carersuk.org
[^4]: https://library.xrguild.org/ai-ethics/ai-hiring-tools-may-be-filtering-out-the-best-job-applicants
[^5]: https://americanstaffing.net/posts/2023/09/07/ai-recruiting-tools
[^6]: https://www.mdpi.com/2673-2688/5/1/19
[^7]: http://gendershades.org
[^8]: https://www.sfgate.com/business/article/How-tech-s-lack-of-diversity-leads-to-racist-6398224.php
[^9]: https://www.theguardian.com/technology/2019/nov/10/apple-card-issuer-investigated-after-claims-of-sexist-credit-checks
[^10]: https://doi.org/10.1145/3375627.3375810
[^11]: https://link.springer.com/article/10.1007/s43681-023-00362-w
[^12]: https://hbr.org/2020/10/to-build-less-biased-ai-hire-a-more-diverse-team
[^13]: https://www.thomsonreuters.com/en-us/posts/legal/ai-enabled-anti-black-bias